Note
Click here to download the full example code
Download metadata from Xeno-Canto to infer species activities¶
The goal of this example is to show how to download metadata from Xeno-Canto to infer species activities. We focus on the activity of european woodpeckers.
Dependencies: To execute this example you need to have installed pandas package.
(from https://woodpeckersofeurope.blogspot.com/2007/11/drumming.html) Woodpeckers of Europe 10 species of woodpecker (Picidae) breed in Europe: 9 resident species and the migratory Wryneck. 8 of these 10 also occur outside Europe, with the distribution of Eurasian Three-toed, White-backed, Lesser Spotted, Great Spotted, Black & Grey-headed Woodpeckers stretching eastwards from the Western Palearctic into Asia, whilst Syrian is found in the Middle East & Asia Minor & Wryneck winters in Africa. The global ranges of Green & Middle Spotted Woodpeckers are confined to the Western Palearctic.
Eurasian Three-toed : Picoides tridactylus White-backed : Dendrocopos leucotos Lesser Spotted : Dryobates minor Great Spotted : Dendrocopos major Black : Dryocopus martius Grey-headed : Picus canus Syrian : Dendrocopos syriacus Wryneck : Jynx torquilla Green : Picus viridis Middle Spotted : Dendrocoptes medius
# sphinx_gallery_thumbnail_path = './_images/sphx_glr_plot_woodpeckers_drumming_annual_activity.png'
import pandas as pd
import matplotlib.pyplot as plt
import numpy as np
from maad import util
Query Xeno-Canto¶
array with english name and scientific name of all european woodpeckers
data = [['Eurasian Three-toed', 'Picoides tridactylus'],
['White-backed', 'Dendrocopos leucotos'],
['Lesser Spotted', 'Dryobates minor'],
['Great Spotted', 'Dendrocopos major'],
['Black', 'Dryocopus martius'],
['Grey-headed', 'Picus canus'],
['Syrian', 'Dendrocopos syriacus'],
['Wryneck', 'Jynx torquilla'],
['Green', 'Picus viridis'],
['Middle Spotted', 'Dendrocoptes medius']]
creation of a dataframe for the array with species names
df_species = pd.DataFrame(data,columns =['english name',
'scientific name'])
get the genus and species needed for Xeno-Canto
Build the query dataframe with columns paramXXX gen : genus cnt : country area : continent (europe, america, asia, africa) q : quality (q_gt => quality equal or greater than) len : length of the audio file (len_lt => length lower than;
len_gt => length greater than )
type : type of sound : ‘song’ or ‘call’ or ‘drumming’ Please have a look here to know all the parameters and how to use them : https://xeno-canto.org/help/search
Get recordings metadata corresponding to the query
df_dataset = util.xc_multi_query(df_query,
format_time = True,
format_date = True,
verbose=True)
Out:
Loading page 1...
https://www.xeno-canto.org/api/2/recordings?query=Picoides%20tridactylus%20area:europe&page=1
searchTerms ['Picoides', 'tridactylus', 'area:europe']
Keeped metadata for 189 files after formating time
Keeped metadata for 125 files after formating date
Found 1 pages in total.
Saved metadata for 125 files
Loading page 1...
https://www.xeno-canto.org/api/2/recordings?query=Dendrocopos%20leucotos%20area:europe&page=1
searchTerms ['Dendrocopos', 'leucotos', 'area:europe']
Keeped metadata for 138 files after formating time
Keeped metadata for 103 files after formating date
Found 1 pages in total.
Saved metadata for 103 files
Loading page 1...
https://www.xeno-canto.org/api/2/recordings?query=Dryobates%20minor%20area:europe&page=1
Loading page 2...
https://www.xeno-canto.org/api/2/recordings?query=Dryobates%20minor%20area:europe&page=2
searchTerms ['Dryobates', 'minor', 'area:europe']
Keeped metadata for 385 files after formating time
Keeped metadata for 296 files after formating date
Found 2 pages in total.
Saved metadata for 296 files
Loading page 1...
https://www.xeno-canto.org/api/2/recordings?query=Dendrocopos%20major%20area:europe&page=1
Loading page 2...
https://www.xeno-canto.org/api/2/recordings?query=Dendrocopos%20major%20area:europe&page=2
Loading page 3...
https://www.xeno-canto.org/api/2/recordings?query=Dendrocopos%20major%20area:europe&page=3
Loading page 4...
https://www.xeno-canto.org/api/2/recordings?query=Dendrocopos%20major%20area:europe&page=4
Loading page 5...
https://www.xeno-canto.org/api/2/recordings?query=Dendrocopos%20major%20area:europe&page=5
searchTerms ['Dendrocopos', 'major', 'area:europe']
Keeped metadata for 653 files after formating time
Keeped metadata for 483 files after formating date
Found 5 pages in total.
Saved metadata for 483 files
Loading page 1...
https://www.xeno-canto.org/api/2/recordings?query=Dryocopus%20martius%20area:europe&page=1
Loading page 2...
https://www.xeno-canto.org/api/2/recordings?query=Dryocopus%20martius%20area:europe&page=2
Loading page 3...
https://www.xeno-canto.org/api/2/recordings?query=Dryocopus%20martius%20area:europe&page=3
searchTerms ['Dryocopus', 'martius', 'area:europe']
Keeped metadata for 263 files after formating time
Keeped metadata for 198 files after formating date
Found 3 pages in total.
Saved metadata for 198 files
Loading page 1...
https://www.xeno-canto.org/api/2/recordings?query=Picus%20canus%20area:europe&page=1
Loading page 2...
https://www.xeno-canto.org/api/2/recordings?query=Picus%20canus%20area:europe&page=2
searchTerms ['Picus', 'canus', 'area:europe']
Keeped metadata for 79 files after formating time
Keeped metadata for 58 files after formating date
Found 2 pages in total.
Saved metadata for 58 files
Loading page 1...
https://www.xeno-canto.org/api/2/recordings?query=Dendrocopos%20syriacus%20area:europe&page=1
searchTerms ['Dendrocopos', 'syriacus', 'area:europe']
/Volumes/lacie_macosx/github/scikit-maad/maad/util/xeno_canto.py:144: SettingWithCopyWarning:
A value is trying to be set on a copy of a slice from a DataFrame
See the caveats in the documentation: https://pandas.pydata.org/pandas-docs/stable/user_guide/indexing.html#returning-a-view-versus-a-copy
df_dataset['time'][df_dataset.time.str.match('^([0-9])[:]([0-5][0-9])$')] = '0' + df_dataset[df_dataset.time.str.match('^([0-9])[:]([0-5][0-9])$')].time
Keeped metadata for 22 files after formating time
Keeped metadata for 18 files after formating date
Found 1 pages in total.
Saved metadata for 18 files
Loading page 1...
https://www.xeno-canto.org/api/2/recordings?query=Jynx%20torquilla%20area:europe&page=1
Loading page 2...
https://www.xeno-canto.org/api/2/recordings?query=Jynx%20torquilla%20area:europe&page=2
searchTerms ['Jynx', 'torquilla', 'area:europe']
Keeped metadata for 0 files after formating time
Keeped metadata for 0 files after formating date
Found 2 pages in total.
Saved metadata for 0 files
Loading page 1...
https://www.xeno-canto.org/api/2/recordings?query=Picus%20viridis%20area:europe&page=1
Loading page 2...
https://www.xeno-canto.org/api/2/recordings?query=Picus%20viridis%20area:europe&page=2
searchTerms ['Picus', 'viridis', 'area:europe']
Keeped metadata for 32 files after formating time
Keeped metadata for 19 files after formating date
Found 2 pages in total.
Saved metadata for 19 files
Loading page 1...
https://www.xeno-canto.org/api/2/recordings?query=Dendrocoptes%20medius%20area:europe&page=1
Loading page 2...
https://www.xeno-canto.org/api/2/recordings?query=Dendrocoptes%20medius%20area:europe&page=2
searchTerms ['Dendrocoptes', 'medius', 'area:europe']
Keeped metadata for 22 files after formating time
Keeped metadata for 17 files after formating date
Found 2 pages in total.
Saved metadata for 17 files
Creation of a dataframe with the number of files per species per 30mins¶
Using the metadata collected from Xeno-Canto, we create a new dataframe containing the number of files per species and per time slot (30 mins). The goal is to create a dataframe with diel pattern of activity for all species with a time resolution of 30 mins.
# make a copy of the dataset to avoid any modification of the original dataset
df = df_dataset.copy()
# remove all rows where data is missing (NA)
df.dropna(subset=['time'], inplace=True)
# Convert time into datetime
df['time'] = pd.to_datetime(df['time'], format="%H:%M")
New dataframe with the number of audio files per time slot. The period of the time slot is 30 min
df_count = pd.DataFrame()
list_species = df['en'].unique()
for species in list_species :
df_temp = pd.DataFrame()
df_temp['count'] = df[df['en']==species].set_index(['time']).resample('30T').count().iloc[:,0]
df_temp['species'] = species
df_count = df_count.append(df_temp)
# create a column with time only
df_count['time'] = df_count.index.strftime('%H:%M')
Creation of a dataframe with the number of files per species per week¶
Using the metadata collected from Xeno-Cant, we create a new dataframe containing the number of files per species and per week. The goal is to create a dataframe with annual pattern of activity for all species with a week (7 days) resolution.
# make a copy of the dataset to avoid any modification of the original dataset
df = df_dataset.copy()
# remove all rows where data is missing (NA)
df.dropna(subset=['week'], inplace=True)
New dataframe with the number of audio files per week
df_week_count = pd.DataFrame()
list_species = df['en'].unique()
for species in list_species :
df_temp = pd.DataFrame()
df_temp['count'] = df[df['en']==species].set_index(['week']).index.value_counts()
df_temp['species'] = species
df_week_count = df_week_count.append(df_temp)
# create a column with time only
df_week_count["week"] = df_week_count.index
Display a heatmap of diel activity¶
make a copy of the dataset to avoid any modification of the original dataset
df = df_count.copy()
find the number of counts that corresponds to 50% of the counts
for species in list_species:
# find the threshold value
count_50_threshold = df[df_count['species']==species]['count'].sum()*(0.50)
# extract the counting value of the category
aa = df[df_count['species']==species]['count'].values
# sort the counts (ascending)
aa.sort()
# reverse the order (descending)
aa = aa[::-1]
# find the index where the cumulative sum of the count is higher
idx = np.where(aa.cumsum() >= count_50_threshold)[0]
aa[idx[0]]
df.loc[(df_count['species'] == species) & (df['count']< aa[idx[0]]), 'count'] = 0
df.loc[(df_count['species'] == species) & (df['count']>=aa[idx[0]]), 'count'] = 1
Display the heatmap to see when (time of the day) the woodpeckers are active. Woodpeckers are the most active during the morning, between 6:00am till 10:00am.
df = df.pivot( 'species', 'time', "count")
df = df.fillna(0)
# plot figure
fig = plt.figure(figsize= (11,2.5))
ax = fig.add_subplot(111)
ax.imshow(df, aspect="auto", interpolation="None", cmap="Set1_r")
# Major ticks
ax.set_xticks(np.arange(0, len(list(df)), 1))
ax.set_yticks(np.arange(0, len(df.index), 1))
# Labels for major ticks
ax.set_xticklabels(list(df),
fontsize=9,
rotation=90)
ax.set_yticklabels(df.index,
fontsize=9)
# Minor ticks
ax.set_xticks(np.arange(-0.5, len(list(df)), 1), minor=True)
ax.set_yticks(np.arange(-0.5, len(df.index), 1), minor=True)
# Gridlines based on minor ticks
ax.grid(which='major', color='w', linestyle='-', linewidth=0)
ax.grid(which='minor', color='w', linestyle='-', linewidth=1)
fig.tight_layout()
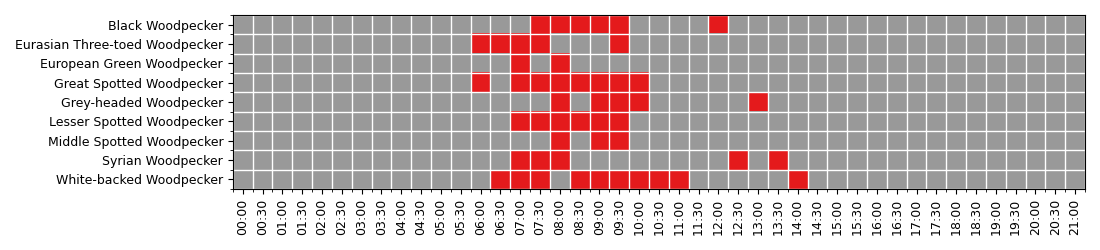
Display a heatmap of annual activity with week resolution¶
make a copy of the dataset to avoid any modification of the original dataset
df = df_week_count.copy()
create a new dataframe with the normalized number of audio files per week
Display the heatmap to see when (annually) the woodpeckers are active. Woodpeckers are the most active during the winter and beginning of spring (Februrary to April).
df = df.pivot( 'species', 'week', "count")
df = df.fillna(0)
# plot figure
fig = plt.figure(figsize= (11,2.5))
ax = fig.add_subplot(111)
ax.imshow(df, aspect="auto", interpolation="None", cmap="Reds")
# Major ticks
ax.set_xticks(np.arange(0, len(list(df)), 1))
ax.set_yticks(np.arange(0, len(df.index), 1))
# Labels for major ticks
ax.set_xticklabels(list(df),
fontsize=9,
rotation=90)
ax.set_yticklabels(df.index,
fontsize=8)
# Minor ticks
ax.set_xticks(np.arange(-0.5, len(list(df)), 1), minor=True)
ax.set_yticks(np.arange(-0.5, len(df.index), 1), minor=True)
# Gridlines based on minor ticks
ax.grid(which='major', color='w', linestyle='-', linewidth=0)
ax.grid(which='minor', color='w', linestyle='-', linewidth=1)
# add the title of the x-axis
ax.set_xlabel("week number")
fig.tight_layout()
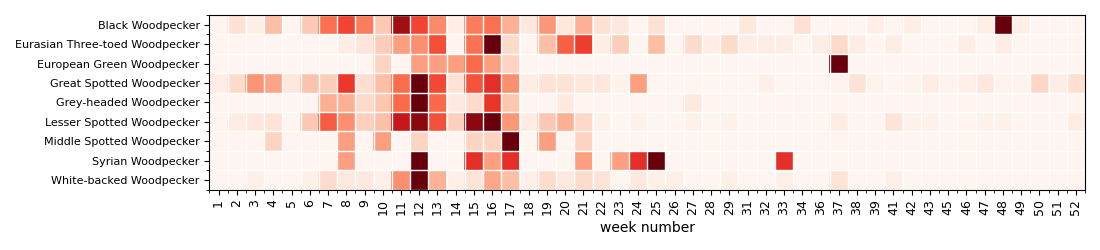
Total running time of the script: ( 1 minutes 35.701 seconds)